Interval Regression using Bayesian Inference
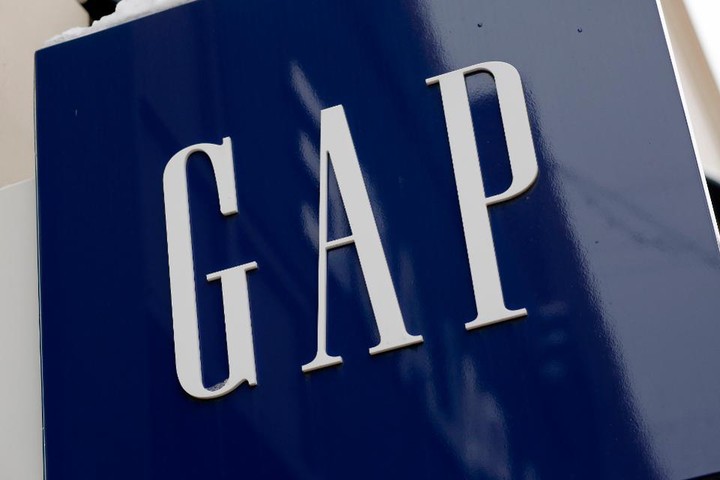
In Fall 2019, I was working with Prof.Vats and Prof. Arshad Rahman (in Economics department) on developing Bayesian models for interval data. I was introduced to interval regression in my summer project on Bayesian estimation of Weibull distribution while reading Prof.Kundu’s paper on interval-censored data with Weibull lifetime. I researched on various paradigms that solve interval regression, covering quantile regression (QR), meta-heuristic algorithms, information theory, convex analysis, and set arithmetic linear models. I found QR to be a promising approach as traditional QR settings can extend to interval data in the following way - we can modify the quantile loss function to have three parts based on the partition by the interval endpoints, as opposed to a binary partition by traditional QR, with certain added assumptions. The change is that the dot product of parameters and covariates lying in the interval has a zero loss as the dependent variable can lie anywhere in the interval. The assumptions can be modelled in a Bayesian setting with appropriate priors. As part of my project, I built Bayesian frameworks for various paradigms of interval regression.