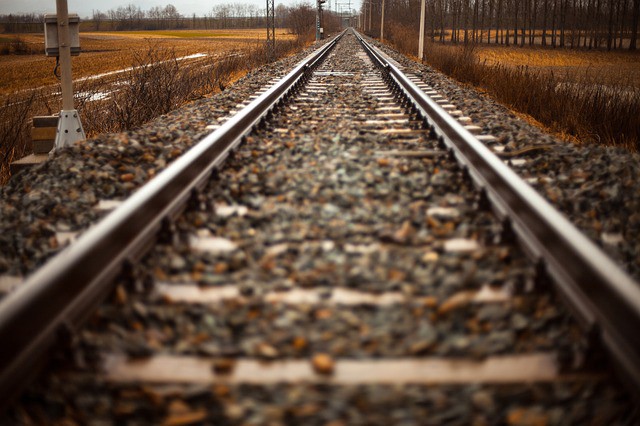
Sufficient research has been done on estimating the asymptotic covariance matrix in a Markov chain central limit theorem for applications in Markov chain Monte Carlo (MCMC). However, almost all of it, including the efficient batch means (BM) estimator, focuses on a single-chain MCMC run. We demonstrate that simply averaging covariance matrix estimators from different chains (average BM) can yield critical underestimates of the variance in small sample sizes, especially for slow mixing Markov chains. We propose a multivariate replicated batch means (RBM) estimator that utilizes information across all chains in order to estimate the asymptotic covariance matrix, thereby correcting for the underestimation. Under weak conditions on the mixing rate of the process, strong consistency of the RBM estimator follows from the strong consistency of the BM estimators. Further, we show that the large-sample bias and variance of the RBM estimator mimics that of the average BM estimator. Thus, for large MCMC runs, the RBM and average BM yield equivalent performance, but for small MCMC runs, the RBM estimator can be dramatically superior. This superiority is demonstrated through a variety of examples, including a two-variable Gibbs sampler for a bivariate Gaussian target distribution. Here we obtain a closed-form expression for the asymptotic covariance matrix of the Monte Carlo estimator, a result vital in itself, as it allows for benchmarking implementations in the future.