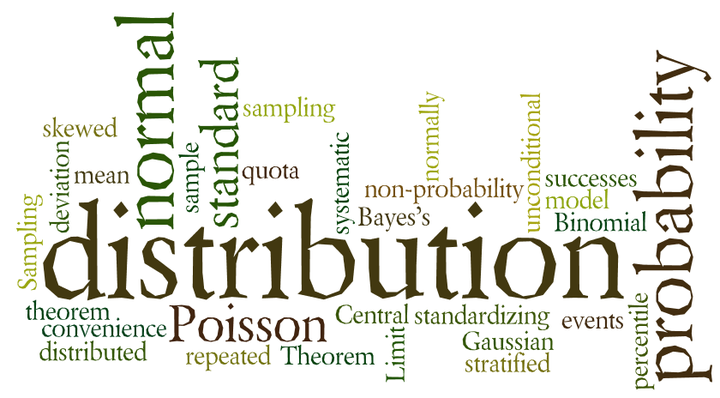
In the summer of 2019, I (in a team of 2) was working with Prof.Kundu on developing Bayesian methodologies for parameter estimation of a 3-Weibull distribution \cite{weibull}. Maximum likelihood estimators are inconsistent and inefficient for this problem when the shape parameter is less than one due to the likelihood function exploding to infinity. However, the Bayesian setting with gamma priors solves this problem as the posterior breaks into a product of proper densities, which is convenient for MCMC sampling. We found the posterior to be log-concave and similar to a gamma distribution and, thus, approximated the parameters and confidence intervals using this observation. Our Bayesian implementation reduced the mean square errors significantly as compared to the maximum likelihood method.